Learn how generative AI works, its key technologies, and its impact on various industries. Discover applications in art, healthcare, and more.
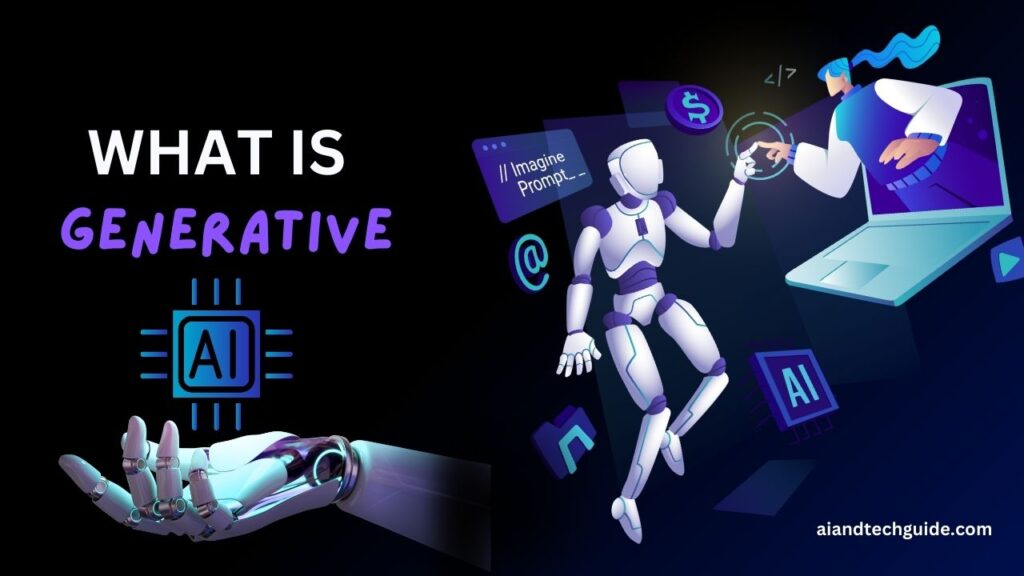
Generative AI: Shaping the Future of Artificial Intelligence
Generative AI is transforming the way we interact with technology, bringing creativity and innovation to the forefront. From creating art and music to advancing medicine and education, this powerful technology is reshaping industries worldwide. In this blog, we’ll explore what generative AI is, how it works, its applications, and its implications for the future.
What Is Generative AI?
Generative AI is a subset of artificial intelligence focused on creating new content, such as images, text, music, or even video, by learning patterns from existing data. Unlike traditional AI, which is primarily reactive, generative AI can innovate, making it a game-changer in creative and problem-solving fields.
The Evolution of Artificial Intelligence
- From Narrow AI to Generative AI
Artificial intelligence has come a long way from performing simple, rule-based tasks to take off human-like creativity. Generative AI represents the next step, empowering machines to produce outputs that were once considered uniquely human.
How Generative AI Works
Generative AI operates by learning patterns and structures from existing data and then using that knowledge to create new, unique outputs. This process involves several key technologies and methodologies:
Neural Networks and Deep Learning
Generative AI is powered by neural networks, a type of machine learning model designed to mimic the way the human brain processes information. Deep learning, a subset of machine learning, uses multiple layers of these neural networks to analyze and interpret complex patterns in large datasets.
For example, a neural network trained on thousands of images can learn to identify features like shapes, textures, and colors, enabling it to generate entirely new images based on what it has learned.
Key Technologies Driving Generative AI
Generative Adversarial Networks (GANs)
GANs are a popular framework in generative AI, consisting of two neural networks:
- The Generator creates new data based on learned patterns.
- The Discriminator evaluates the generated data and determines if it resembles the original dataset.
These two networks work together in a feedback loop, with the generator continuously improving its outputs until they are indistinguishable from real data. This technology powers applications like creating realistic images, deepfakes, and more.
Transformer Models
Transformer models, such as OpenAI’s GPT (Generative Pre-trained Transformer), have revolutionized natural language processing (NLP). They excel at understanding context and generating coherent text by analyzing relationships between words in a sequence.
For instance, a transformer model can generate essays, write poetry, or even simulate human-like conversations. These models rely on massive datasets and advanced algorithms to produce outputs that feel natural and relevant.
The Role of Training Data
Generative AI relies heavily on high-quality training data. The data must be diverse, accurate, and representative of the task at hand. For instance:
- Images: Training a GAN to generate artwork requires a dataset of high-quality images with varying styles.
- Text: A transformer model needs vast amounts of written content to generate meaningful and contextually accurate text.
The Process in Action
- Data Input: Generative AI models are trained on massive datasets, such as text, images, or audio.
- Pattern Learning: The model identifies and learns the relationships within the data.
- Content Generation: Once trained, the model uses what it has learned to produce new, original outputs.
Applications of Generative AI Across Industries
- Art and Design: AI-Powered Creativity
Generative AI tools, such as AI Phocraft DALL-E and Stable Diffusion, are enabling artists to create stunning visuals. These tools can transform simple text prompts into intricate artworks, redefining digital creativity.
- Content Creation: Writing and Beyond
Blog writing, script drafting, and even generating personalized emails have become easier with generative AI. Tools like ChatGPT allow businesses to automate content creation while maintaining quality.
- Music and Audio: AI Composers
Musicians embrace AI to compose melodies, enhance audio tracks, and even recreate iconic styles. AI platforms like Amper Music and AIVA are changing the way music is created.
- Healthcare: Transforming Drug Discovery
Generative AI accelerates drug discovery by predicting molecular structures and simulating medical experiments. This technology is helping researchers develop life-saving treatments faster.
- Gaming and Entertainment
Video game developers use generative AI to design characters, create immersive storylines, and build dynamic game environments, enhancing player experiences.
Popular Generative AI Tools and Platforms
- Tools for Artists
AI art generators like MidJourney and NightCafe have become essential tools for designers and creators, offering a unique way to explore creative possibilities, generate inspiration, and produce digital assets with minimal effort.
Why These Platforms Are Popular
- Ease of Use
Both platforms are user-friendly, enabling anyone, regardless of technical skill, to generate high-quality art using simple prompts. - Diverse Styles and Outputs
- MidJourney excels in producing highly detailed and surreal visuals, making it a favorite for conceptual art and imaginative designs.
- NightCafe allows customization through different algorithms and styles, making it versatile for various creative needs.
- Time Efficiency
These tools dramatically reduce the time it takes to create art, enabling rapid prototyping or visualization of ideas. - Cost-Effective
Instead of hiring artists or purchasing expensive software, creators can access affordable subscriptions or free trials to produce professional-grade art. - Inspiration Source
These platforms help overcome creative blocks by generating fresh ideas, offering new perspectives, and experimenting with artistic styles.
- Platforms for Coders
For developers, platforms like Codex offer AI-powered coding assistance, making programming faster and more efficient.
Ethical Considerations of Generative AI
Copyright and Intellectual Property
Generative AI often uses existing data to create new outputs, raising questions about copyright infringement and ownership of AI-generated content.
Misinformation and Disinformation
AI-generated deepfakes and fabricated content can mislead audiences, emphasizing the need for regulatory frameworks.
Bias and Fairness
Generative AI systems can perpetuate biases present in their training data, leading to unfair outcomes. Addressing these biases is critical for responsible AI development.
Challenges in Generative AI Development
Here are five expanded challenges in generative AI development:
1. Data Bias and Diversity
Generative AI relies heavily on training data, but biases in these datasets can lead to skewed or discriminatory outputs. For example, underrepresentation of certain groups can result in content that marginalizes or stereotypes them. Addressing these biases requires extensive preprocessing and curating of data, which is time-consuming and resource-intensive.
2. High Computational Requirements
Training state-of-the-art models like GPT or GANs demands enormous computational resources, including high-performance GPUs and vast amounts of memory. This not only makes development costly but also raises environmental concerns due to the significant carbon footprint associated with training large models.
3. Ethical Misuse
Generative AI has a dual-use problem: while it can create beneficial applications, it can also be used maliciously. For instance, deepfake technology can produce realistic but fake images or videos, enabling fraud, blackmail, or political misinformation. Ensuring responsible use is a critical challenge for developers and policymakers.
4. Quality Control and Reliability
Generative AI models often struggle to ensure the accuracy and reliability of their outputs. They can produce “hallucinations”—plausible-sounding but incorrect or fabricated information. This limits their application in fields requiring high accuracy, such as healthcare or legal services, without robust human oversight.
5. Regulatory and Governance Challenges
The rapid pace of AI development has outstripped regulatory efforts, leaving developers to operate in an uncertain legal and ethical landscape. Questions around intellectual property, accountability for harmful outputs, and user privacy remain unresolved, complicating widespread adoption and trust in generative AI systems.
Future Trends in Generative AI
- AI-Powered Creativity
As generative AI evolves, it is expected to become a more collaborative tool for artists, designers, and innovators, pushing the boundaries of human imagination.
- Education and Personalized Learning
Generative AI has the potential to create tailored learning experiences, from personalized quizzes to adaptive teaching materials.
- Virtual Reality Integration
Combining generative AI with virtual reality could lead to groundbreaking developments in immersive storytelling and interactive environments.
The Debate Around Generative AI
Generative AI has emerged as one of the most transformative yet controversial technologies of the modern era. While its potential to revolutionize industries and enhance creativity is undeniable, it also raises ethical, societal, and technical concerns. Here’s an exploration of the debate surrounding generative AI:
Pros: The Promise of Generative AI
- Creative Innovation
Generative AI enhances creative fields by generating text, images, music, and designs. Tools like DALL·E, ChatGPT, and others enable artists, writers, and designers to prototype ideas faster, often pushing the boundaries of creativity. - Automation and Efficiency
Generative AI automates repetitive and time-consuming tasks, such as content creation, marketing copy generation, and even code development. This allows professionals to focus on higher-value tasks. - Accessibility
AI tools democratize access to skills and expertise, empowering individuals without specialized training to create professional-grade content, from graphics to video editing. - Scientific Advancements
Generative AI aids in drug discovery, medical imaging, and simulating scientific models, potentially accelerating breakthroughs in healthcare and beyond. - Personalization
From customized shopping experiences to tailored educational content, generative AI enhances personalization, improving user satisfaction and engagement.
Cons: The Concerns of Generative AI
- Misinformation and Deepfakes
The ability of generative AI to produce realistic yet fake content, such as deepfake videos or false articles, poses a significant threat to trust and truth in the digital age. - Job Displacement
Automation of creative and analytical tasks threatens traditional jobs in industries like content creation, design, customer service, and even software development. - Bias and Ethical Concerns
Generative models often reproduce biases present in their training data, leading to discriminatory outputs. Additionally, ethical dilemmas arise around the use of AI for malicious purposes. - Intellectual Property Issues
The use of copyrighted materials in training datasets raises questions about ownership and fair use, with creators expressing concerns about unauthorized replication of their work. - Environmental Impact
Training and operating large generative models require significant computational resources, leading to a high carbon footprint that exacerbates environmental issues.
How to Get Started with Generative AI
- Beginner Resources
Start by exploring free online courses and tutorials on machine learning and AI. Platforms like Coursera and Khan Academy offer beginner-friendly resources.
- Advanced Learning
For a deeper understanding, explore frameworks like TensorFlow, PyTorch, and OpenAI’s tools. Experiment with coding and building basic models to gain hands-on experience.
Conclusion: Generative AI
Generative AI is more than a technological advancement; it’s a tool for reimagining creativity, problem-solving, and innovation. While it brings immense opportunities, it also demands responsible use and ethical considerations. As we embrace generative AI, the challenge lies in balancing its potential with its impact on society.